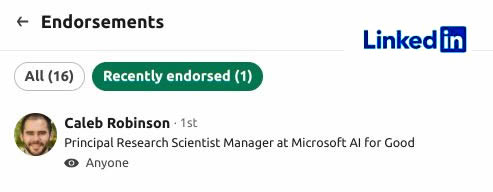
AI for Good, Principal Research Science Mgr MSFT, recommends Brian Hamlin for OpenSource
never hurts to get recognition from leaders in the field of GeoAI
AI for Good, Principal Research Science Mgr MSFT, recommends Brian Hamlin for OpenSource
never hurts to get recognition from leaders in the field of GeoAI
It is Official
https://knowingmachines.org/about
Well-known proverbs in Finnish and English (credit: Wikipedia)
Time heals wounds
Aika parantaa haavat
Not two without a third.
Ei kahta ilman kolmatta
No boneless meat, no headless fish.
The name doesn’t make a man worse, unless he names it.
Not learning to pour into a ditch.
No smoke without fire.
Crying for joy.
Hidden gold has no price.
At the end, acknowledgment stands.
What you leave behind, you find in front of you.
Both lie down and cheat.
The forest answers as it is shouted.
Own land strawberry, other land blueberry.
An apple a day keeps the doctor away.
Learn age all.
Better a laundry in a day than ten branches.
Pot boiler, black side with each.
The brave rock eats.
That dog doesn’t go where the fish goes.
It is best to laugh, who last laughs.
Catch the moon at the bottom of the home
An empty sack cannot stand.
One fool asks more than ten wise men can answer.
One rotten apple spoils the whole basket.
—
.
Ei luutonta lihaa, ei päätöntä kalaa.
Ei nimi miestä pahenna, ellei mies nimeä.
Ei oppi ojaan kaada.
Ei savua ilman tulta.
Itku pitkästä ilosta.
Kätketyllä kullalla ei ole hintaa.
Lopussa kiitos seisoo.
Minkä taakseen jättää, sen edestään löytää.
Niin makaa kuin petaa.
Niin metsä vastaa kuin sinne huudetaan.
Oma maa mansikka, muu maa mustikka.
Omena päivässä pitää lääkärin loitolla.
Oppia ikä kaikki.
Parempi pyy pivossa kuin kymmenen oksalla.
Pata kattilaa soimaa, musta kylki kummallakin.
Rohkea rokan syö.
Se koira älähtää, johon kalikka kalahtaa.
Se parhaiten nauraa, joka viimeksi nauraa.
Sitä kuusta kuuleminen, jonka juurella asunto.
Tyhjä säkki ei pystyssä pysy.
Yksi hullu kysyy enemmän kuin kymmenen viisasta ehtii vastata.
Yksi mätä omena pilaa koko korin.
Recently, a FOSS4G event was held in Prizren, in the Balkans. Here are some research resources:
The Government of Canada (GC) recently published a broad strategy overview on the topic of satellite Earth Observation. The document -LINK- is public-facing and emphasizes the “three-Rs” of Resourceful Resilient Ready; a companion document not reviewed here, is written from a National Defence (sic) point of view, called Strong Secure Engaged. This pair, Defense and Sustainability, appear frequently in national strategy documents in the age of Climate Change.
This twenty page document, filled with impressive color photos of the world as viewed from above, is indeed a “green” document and contains many themes familiar in the broad sustainability movement and its vocabulary. The phrase Open Data is mentioned several times, but the acronym FAIR, common in academic circles, does not appear. Ironically, this document gives some passing support to the “economic value of open data” yet recently in the United States under the previous Presidency, weather data, EPA publications and most anything to do with Climate was made drastically less public, while engaging private business partnerships, allegedly to make better use of the resources. This Canadian GC document mentioned the European Union several times, but seldom the USA, its geographic neighbor and long-term military partner. Also new to me was the English-only presentation. The few GC documents I had reviewed in the past always had French language prominently along with English.
When remote sensing and Climate are the topic, flooding and sea level are always included, and this document is no exception. Flood and coastline analysis in remote sensing is a specialty, and I won’t be including much on that here. Another obvious aspect of remote sensing in Canada is that Canada is massive, complex and difficult to map for many reasons. If anyone needs remote sensing from satellites, it is Canada. In fact, we are informed that historically, Canada was the third nation in the world to operate satellites, presumably after the USA and USSR, in the early 1960s. Canada is part of the Arctic Council today, where some members are active competitors for natural resources and shipping. In plain talk, remote sensing has been used from the beginning to watch and measure the economic activity of competitors, and that remains a reliable revenue stream for this expensive endeavor to this day. Included here are details on some important and ongoing environmental sensor missions, including ozone layer and greenhouse gas monitoring, now more important than ever.
Glossy diagrams allegedly showing the benefits of alliance between Academic, Industry and Government programs, appeared to be shallow and relatively low-quality contributions, included for the positive message perhaps, but lacking important details and over-simplifying real life activity, effects and reach of these constantly changing partnerships. The document is an overview and is somewhat lacking in hard facts, other than the existence of showcased sensor satellite missions. Like similar green documents from California, a partnership with First Nations is extensively featured in one section, including education and training for traditionally underserved communities.
From the technology side, one might split topics between “Industry Support” and “Health & Safety.” Industry meaning existing economic activity, agriculture, resources flows, effects on jobs and the like, with Health and Safety including government functions like the monitoring of pest-born disease, water quality, and wildfires. None of these topics are new, especially in light of Canada being an early space pioneer, but the rate, quality and handling of satellite-based remote sensing data is new. Over the decades, it has to be said that previous specialized heavyweights such as High Performance Computing (HPC) have been eclipsed and I would say even embarrassed by the sheer capacity of Google, and more recently Amazon Web Services cloud computing. Despite public posturing, it is rumored that even the European Union Space Agency (ESA) itself uses AWS behind the scenes for its cost and performance. Google, AWS and others have routinely implemented Machine Learning systems on data flows for commercial purposes, techniques that remain mysterious and out of reach today even for established and well-connected companies and government. The ill-defined and somedays dubious term Artificial Intelligence appears in many consumer-oriented marketing material and the buzzwords of business plan promotion, but it is safe to say that we are in early days there still.
Wrapping up, it could be said that we are living in an AWS, post-Google world now, with cloud computing backends for exponentially increasing volumes of remote sensing data. Most advanced nations are promoting high tech for competitive reasons, and the Government of Canada presents its case here.